by Michael Bani
6 minutes
How AI is Transforming Personalized Medicine for Better Healthcare Outcomes
We'll explore AI's pivotal role in personalized medicine and shed light on how it enables tailored treatments and improves therapeutic outcomes.
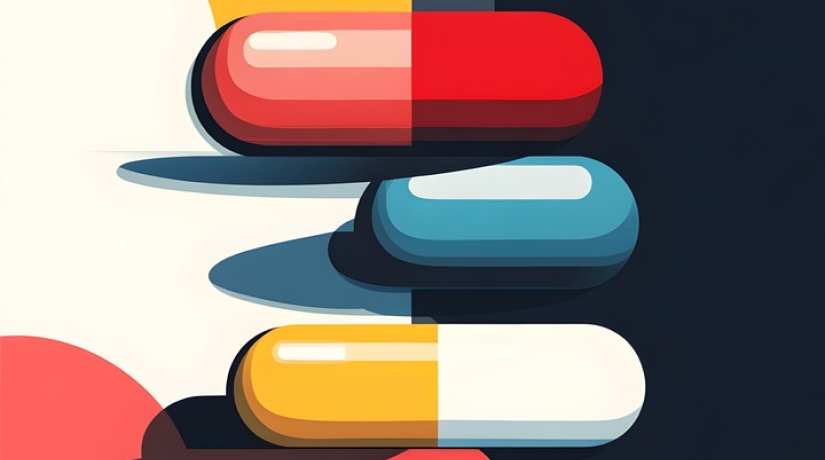
Every one of us is unique when it comes to our genetic profile. A medicine prescribed to one person may not give similar results to another. This is where personalised medicine comes into play. Personalised medicine uses an individual’s genetic profile to make decisions regarding the treatment of a disease, and that is a total game-changer.
With the help of AI, we can analyse large chunks of data and get improved treatment outcomes. In this article, we will see how AI is easing the difficulties associated with traditional healthcare practices, the technologies used in personal medicine and some case studies.
Limitations of Traditional Healthcare Practices
Traditional Healthcare Practices follow a fixed set of rules. These rules are based on the general population and clinical trials and are continuously updated with the latest research and best practices. Doctors diagnose and treat similar symptoms based on these regulations. However, as the world evolves, traditional healthcare practices fall short of treating diseases. Some of the limitations are :
- Almost the same standard diagnostic tests and medications are prescribed based on the existing guidelines.
- Individual conditions and genes can lead to different responses to the same treatment.
- Genetics, lifestyle, environment, and personal preferences influence treatment outcomes for which there is no provision in the traditional approach.
AI Technologies in Personalized Medicine
AI helps provide personalised medications, resulting in better treatments via machine learning, NLP, and deep learning. Let us find out about them in detail.
Machine Learning Algorithms:
Machine learning algorithms analyse and enhance medicine's efficiency, accuracy and personalisation, which results in better treatment. They take data from past cases and improve treatment outcomes.
Deep Learning in Image Analysis :
Deep learning algorithms mainly help radiologists and oncologists diagnose accurately and create personalised treatment plans by doing image analysis. This enables precise interpretation of radiological and MRI scans depending on each individual.
Natural Language Processing (NLP) :
Natural language processing (NLP) extracts data from unstructured clinical notes and identifies the essential details. This results in making informed decisions, which enhances efficiency in personalised medicine.
How is AI transforming personalised treatment approaches?
AI is a boon in personalised medicine, which uses genomics along with AI to tailor treatments that improve outcomes. AI revolutionises healthcare in many ways, from diagnostics to treatment optimisation. It predicts disease progression and responses for highly effective, low-risk interventions.
In Diagnostics
AI can analyse vast amounts of medical images, genetic profiles, and clinical data and provide new information. This is a massive help as it helps identify the early signs of a disease, and a person can start early treatment. It is one of the significant benefits of AI in diagnostics.
AI compiles all the information from different sources and detects subtle signs that may escape the human eye. This results in correct and early detection of disease.
Steady Monitoring and Treatment Optimization
Immediate adjustments can be made in the medication since AI provides steady monitoring and optimisation of treatment. Treatment can be changed by monitoring the patient's situation by analysing patient data and feedback streams. It ensures that therapy remains aligned with the patient's characteristics and goals.
Integrating Multimodal Data Sources
AI in personalised medicine is advancing by integrating multimodal data sources. AI algorithms can then analyse diverse data types, such as medical images, genomic data, clinical notes, and wearable sensor data. AI synthesises information from multiple sources and fully understands an individual's unique health. This provides precise medication options.
Increasing Patient Engagement and Adherence
With AI techniques like intelligent monitoring systems and interactive applications, people can be participants in their healthcare journey. It provides customised feedback, reminders, and educational resources so that people can maintain their routines.
Streamlining The Work Of Doctors
AI empowers clinicians to streamline their workflow in all departments, from diagnostic assistance to treatment planning and monitoring. AI-driven analytics allow doctors to make more informed decisions, optimise resource allocation, and provide personalised care for individuals.
Genomic Sequencing and Analysis
Genomic sequencing and Analysis uses AI to analyse a person’s genetic build. This helps AI identify genetic variants for disease susceptibility, treatment response and adverse drug reactions to provide personalised treatment plans based on a patient's genetic profile, optimise therapeutic outcomes and minimise risks.
Power of AI In Predictive Analytics and Risk Stratification
Predictive analytics can make use of AI techniques to detect disease progression. Predictive models can divide patients based on demographic information, medical history, and biomarkers to achieve better outcomes.
Case Studies and Success Stories
Let us have a look at some case studies where AI has helped in providing personalised treatment:
1. GNS Healthcare's REFS platform: The REFS platform uses AI to analyse genomic, clinical, and real-world evidence and model complex biological systems, which results in better outcomes. It provides personalised treatment for chronic diseases like diabetes and multiple sclerosis.
2. DeepMind's AlphaFold: DeepMind's AlphaFold predicts protein structures, which is essential for drug discovery and personalised medicine. AlphaFold's breakthrough performance in the Critical Assessment of Structure Prediction (CASP) competition has shown promising results in accelerating drug development.
3. IBM Watson for Oncology: IBM Watson, a cognitive computing system in oncology, analyses vast datasets. In a study conducted in India, Watson for Oncology recommended treatment plans for breast cancer patients, which were accurate.
Current Challenges
Despite AI's benefits in personalised medicine, the field is full of challenges which must be addressed to reap the full advantage.
Data Governance Frameworks
The chief challenge is strong data governance frameworks to ensure the safety of patient data and its ethical use, as AI algorithms use vast amounts of sensitive information to generate insights.
Standardisation and Interoperability
The lack of standardisation and difficulty transferring data back and forth makes integrating AI technologies into the healthcare system difficult. Efforts should be made so that a seamless data exchange is possible, which results in better outcomes.
Addressing Biases in Healthcare Data
AI algorithms based on biased datasets may worsen the treatment, especially for the vulnerable population. All the efforts should be made to balance training data, mitigate algorithmic biases, and ensure that AI methods are suited for all.
Infrastructure and Resource Constraints
Constraints like High power, storage, and specialised hardware costs must be taken care of so that AI can bring an impactful change in personalised medicine.
Regulatory And Ethical Issues
With AI changing personalised medicine, ethical questions must be addressed, such as how the algorithm works, AI misuse, and patient consent.
The Future
The future of personalised medicine is bright with AI by its side.
- Integration of Multimodal Data Sources: As healthcare data grows from sources like electronic health records and wearable devices, AI algorithms, including machine learning and deep learning, unify these data for insights so that personal treatment plans can be provided to patients.
- Explainable AI Models: AI analyses complex data and makes predictions based on this data. However, they are not entirely trustworthy. Explainable AI techniques aim to provide interpretable explanations for AI decisions, increasing transparency and building trust.
- Emphasis on Clinical Translation: New AI algorithms are needed to use AI in personalised medicine at the clinical level.
- Ethical and Regulatory Considerations: As AI in personalised medicines develops, further ethical practices like ensuring data privacy and obtaining patient's consent for their data should be encouraged to succeed.
Conclusion
AI is bringing a massive change in the field of personalised medicine. However, as we move forward, challenges like data privacy and interoperability need to be tackled effectively to use the maximum benefits of AI in this field and improve an individual's quality of life.
FAQs
What are the real-world applications of AI in personalised medicine?
Real-world applications include cancer treatment planning, genetic analysis, personalised medicine use, and chronic disease management.
How does AI enhance preventive care in personalised medicine?
With the help of AI tools, one can understand how much a person is susceptible to a particular disease and can take preventive measures.
What are the ethical considerations of using AI in personalised medicine?
Ethical considerations include data privacy, algorithmic bias, informed consent, and ensuring that AI benefits are accessible to all patients.